篇名:A Probability Density Function Generator Based on Neural Networks
作者:Chi-Hua Chen(陈志华), Fangying Song*, Feng-Jang Hwang, Ling Wu
来源:Physica A
年份:2019
DOI: https://doi.org/10.1016/j.physa.2019.123344
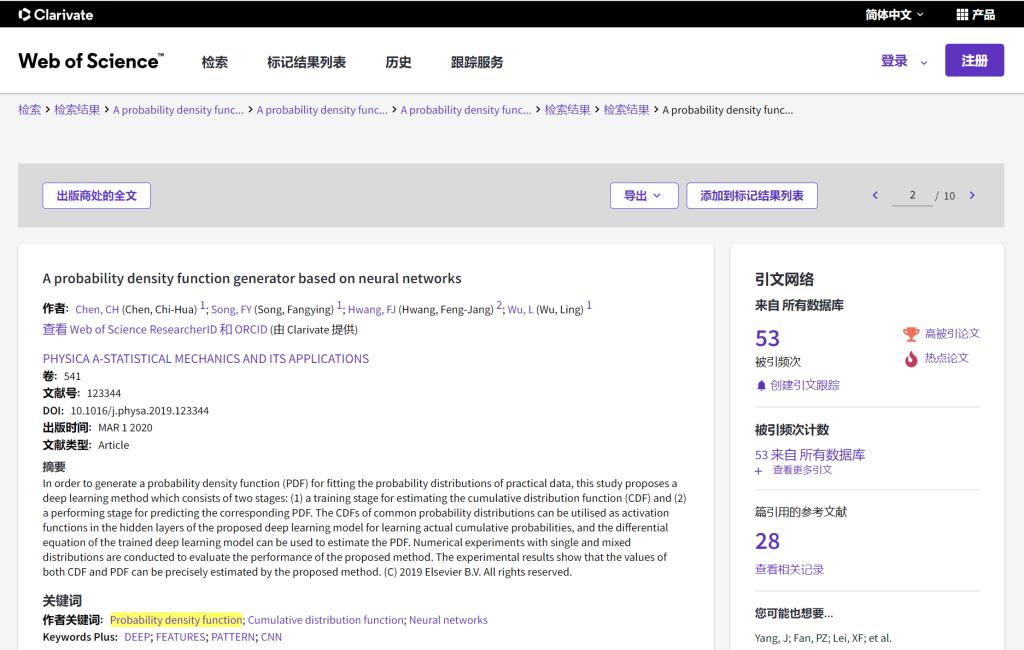
文章摘要:
In order to generate a probability density function (PDF) for fitting the probability distributions of practical data, this study proposes a deep learning method which consists of two stages: (1) a training stage for estimating the cumulative distribution function (CDF) and (2) a performing stage for predicting the corresponding PDF. The CDFs of common probability distributions can be utilised as activation functions in the hidden layers of the proposed deep learning model for learning actual cumulative probabilities, and the differential equation of the trained deep learning model can be used to estimate the PDF. Numerical experiments with single and mixed distributions are conducted to evaluate the performance of the proposed method. The experimental results show that the values of both CDF and PDF can be precisely estimated by the proposed method.
为了生成用于拟合实际数据概率分布的概率密度函数(PDF),本研究提出了一种深度学习方法,该方法包括两个阶段:(1)用于估计累积分布函数(CDF)的训练阶段和(2)用于预测相应PDF的执行阶段。公共概率分布的CDF可以用作建议的深度学习模型的隐藏层中的激活函数,用于学习实际累积概率,并且可以使用经过训练的深度学习模型的微分方程来估计PDF。通过单分布和混合分布的数值实验对该方法的性能进行了评价。实验结果表明,该方法能够准确估计CDF和PDF的值。
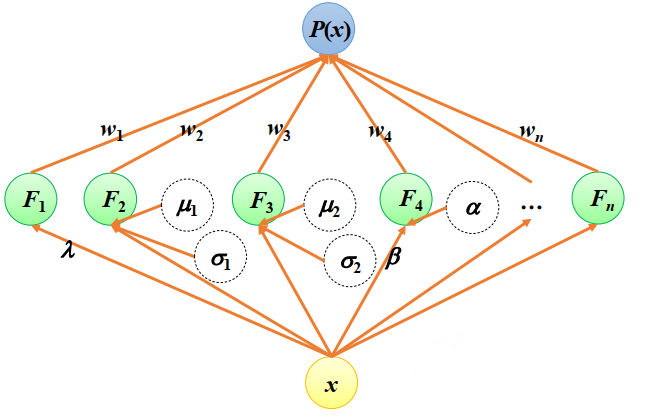