篇名:Attention-based stackable graph convolutional network for multi-view learning
作者:Zhiyong Xu, Weibin Chen, Ying Zou, Zihan Fang, Shiping Wang
年份:2024
DOI:https://doi.org/10.1016/j.neunet.2024.106648
文章摘要:
In multi-view learning, graph-based methods like Graph Convolutional Network (GCN) are extensively researched due to effective graph processing capabilities. However, most GCN-based methods often require complex preliminary operations such as sparsification, which may bring additional computation costs and training difficulties. Additionally, as the number of stacking layers increases in most GCN, over-smoothing problem arises, resulting in ineffective utilization of GCN capabilities. In this paper, we propose an attentionbased stackable graph convolutional network that captures consistency across views and combines attention mechanism to exploit the powerful aggregation capability of GCN to effectively mitigate over-smoothing. Specifically, we introduce node self-attention to establish dynamic connections between nodes and generate view-specific representations. To maintain cross-view consistency, a data-driven approach is devised to assign attention weights to views, forming a common representation. Finally, based on residual connectivity, we apply an attention mechanism to the original projection features to generate layer-specific complementarity, which compensates for the information loss during graph convolution. Comprehensive experimental results demonstrate that the proposed method outperforms other state-of-the-art methods in multi-view semi-supervised tasks.
关键词:
Machine learning Multi-view learning Graph convolutional network Attention mechanism Semi-supervised classification
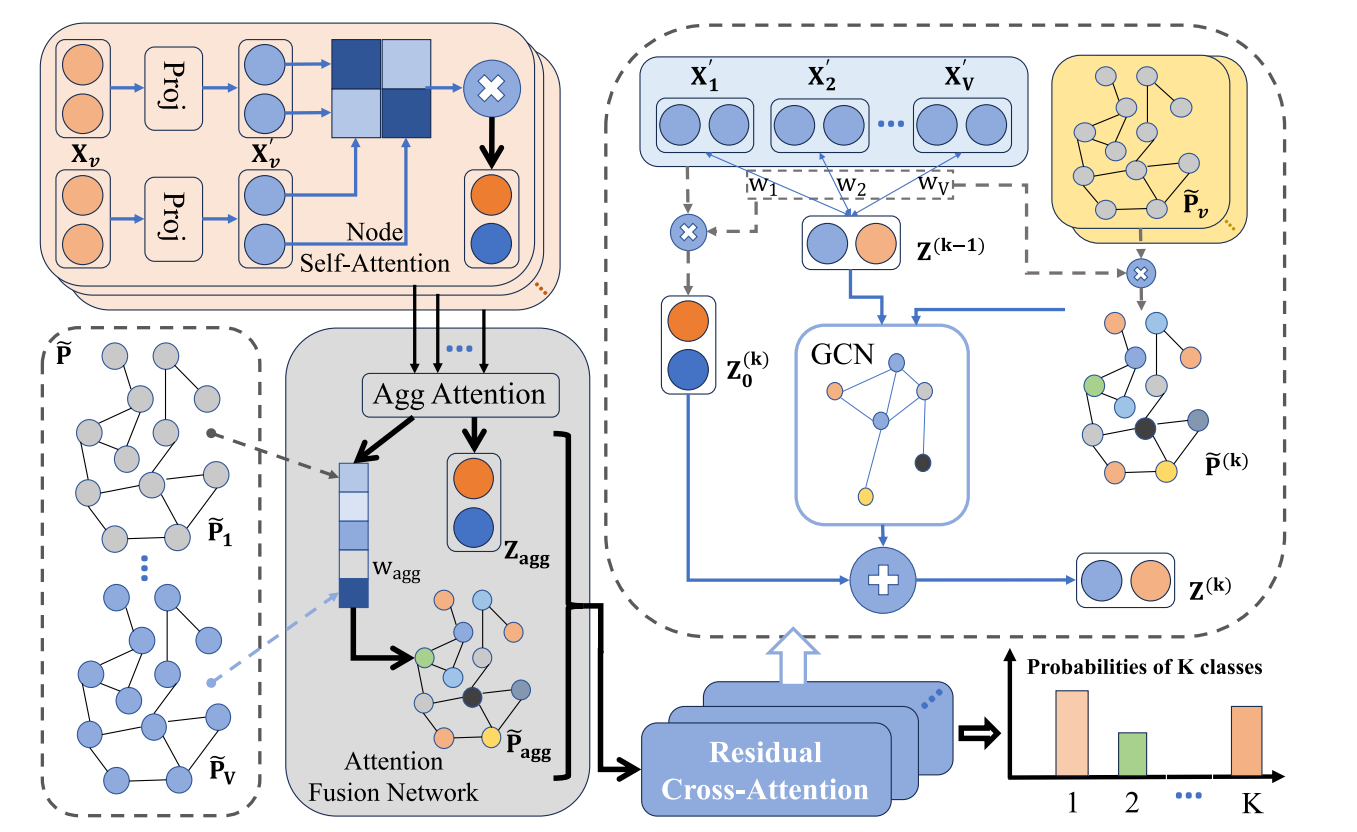