篇名:Multi-view clustering via optimal transport algorithm
作者:Renjie Lin, Shide Du, Shiping Wang, Wenzhong Guo
年份:2023
DOI:https://doi.org/10.1016/j.knosys.2023.110954
文章摘要:
The surge in data with multiple views has propelled significant interest in the domain of multi-view clustering. Unlike conventional single-view data, multi-view data offers a more accurate representation of objects. However, the pivotal challenge remains in the effective categorization of data through feature extraction from multiple views within clustering tasks. Notably, prevailing multi-view clustering algorithms often emphasize the derivation of appropriate view weights, inadvertently sidestepping optimization intricacies. This approach frequently leads to protracted computational time due to the resource-intensive matrix multiplication operations involved in optimization, coupled with the necessity of weight allocation for diverse views. Addressing this, we propose an optimization framework founded on the optimal transport algorithm that operates independently of view weights. A paramount advantage of the optimal transport algorithm lies in its rapid convergence to a closed-form solution. This study diverges from the conventional focus on view weights and centers on the optimization process within clustering algorithms for resolving multi-view challenges. The introduced framework employing the optimal transport algorithm significantly mitigates computational complexity while handling multiple views. Rigorous experimentation duly substantiates the efficacy of the proposed framework in the realm of multi-view clustering. Across publicly available multi-view datasets, our framework exhibits superior performance over existing state-of-the-art algorithms.
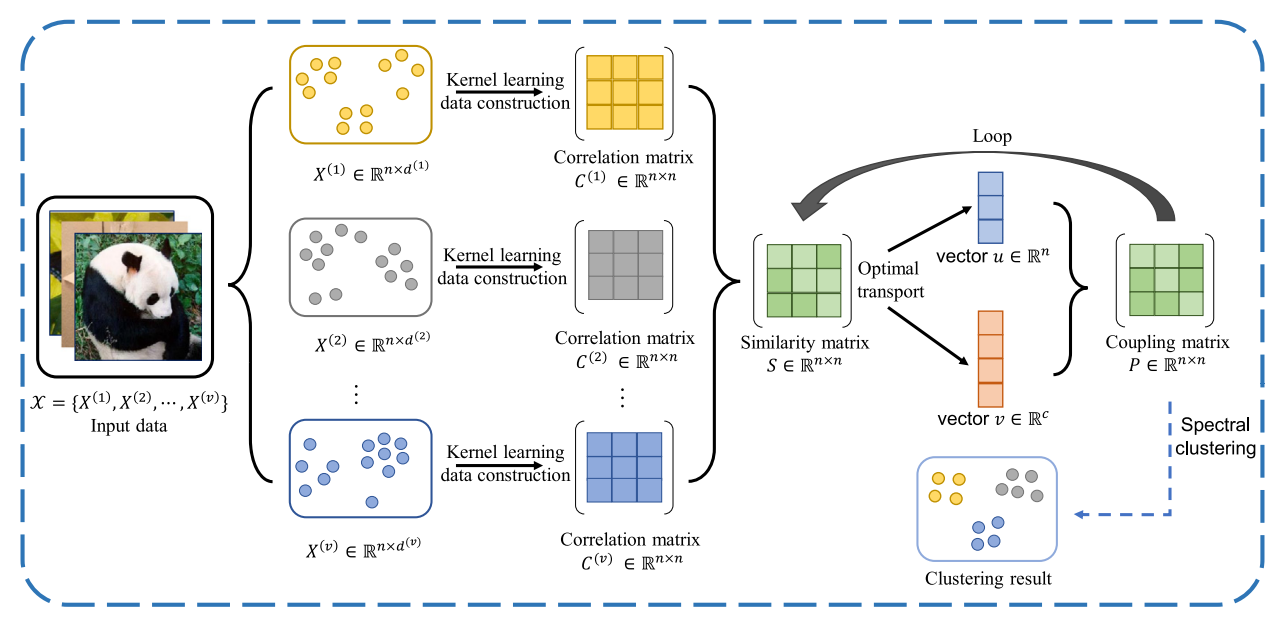