篇名:Graph Convolutional Network with elastic topology
作者:Zhihao Wu, Zhaoliang Chen, Shide Du, Sujia Huang, Shiping Wang
年份:2024
DOI:https://doi.org/10.1016/j.patcog.2024.110364
文章摘要:
Graph Convolutional Network (GCN) has drawn widespread attention in data mining on graphs due to its outstanding performance and rigor theoretical guarantee. However, some recent studies have revealed that GCN-based methods may mine latent information insufficiently owing to the underutilization of the feature space. Besides, the unlearnable topology also significantly imperils the performance of GCN-based methods. In this paper, we conduct experiments to investigate these issues, finding that GCN does not fully consider the potential structure in the feature space, and a fixed topology deteriorates the robustness of GCN. Thus, it is desired to distill node features and establish a learnable graph. Motivated by this goal, we propose a framework dubbed Graph Convolutional Network with elastic topology (GCNet1 ). With the analysis of the optimization for the proposed flexible Laplacian embedding, GCNet is naturally constructed by alternative graph convolutional layers and adaptive topology learning layers. GCNet aims to deeply explore the feature space and employ the mined information to construct a learnable topology, which leads to a more robust graph representation. In addition, a set-level orthogonal loss is utilized to meet the orthogonal constraint required by the flexible Laplacian embedding and promote better class separability. Moreover, comprehensive experiments indicate that GCNet achieves remarkable performance and generalization on several real-world datasets.
关键词:
Graph convolutional networks Semi-supervised classification Learnable topology Orthogonal constraint
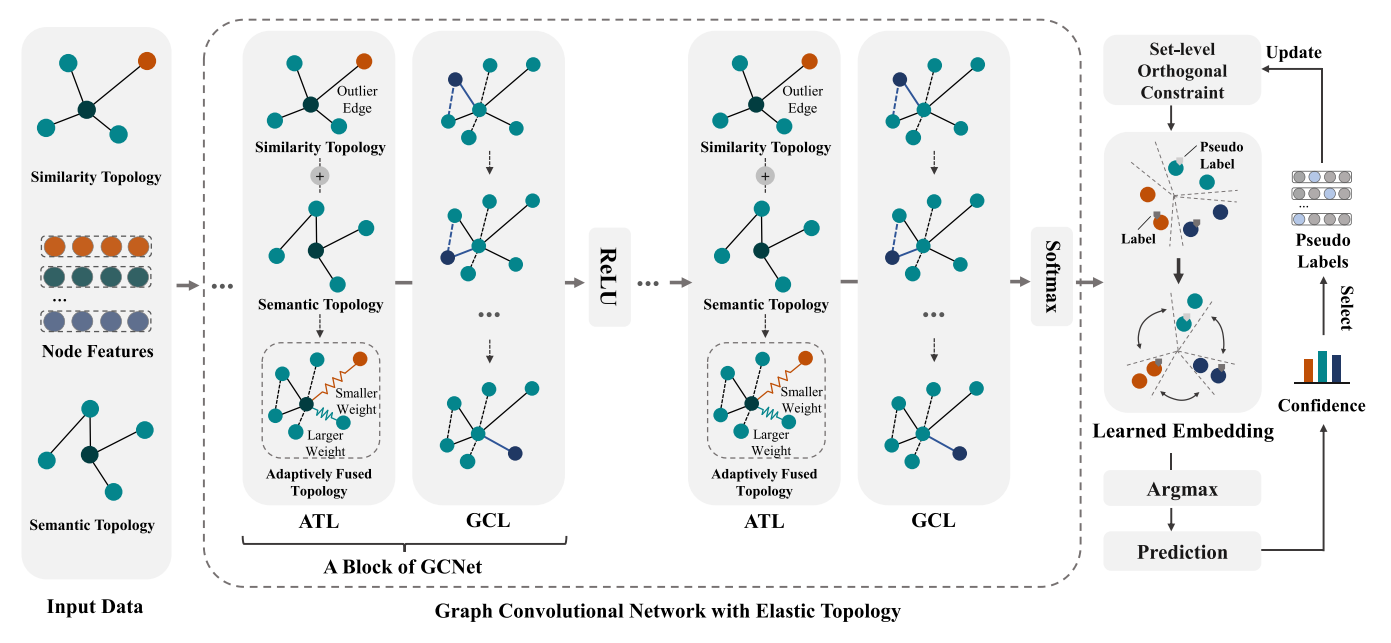