篇名:Representation Learning Meets Optimization-Derived Networks: From Single-View to Multi-View
作者:Zihan Fang , Shide Du , Zhiling Cai , Shiyang Lan , Chunming Wu , Yanchao Tan, Shiping Wang
年份:2024
DOI:https://doi.org/10.1109/TMM.2024.3383295
文章摘要:
Existing representation learning approaches lie predominantly in designing models empirically without rigorous mathematical guidelines, neglecting interpretation in terms of modeling. In this work, we propose an optimization-derived representation learning network that embraces both interpretation and extensibility. To ensure interpretability at the design level, we adopt a transparent approach in customizing the representation learning network from an optimization perspective. This involves modularly stitching together components to meet specific requirements, enhancing flexibility and generality. Then, we convert the iterative solution of the convex optimization objective into the corresponding feed-forward network layers by embedding learnable modules. These above optimization-derived layers are seamlessly integrated into a deep neural network architecture, allowing for training in an end-to-end fashion. Furthermore, extra view-wise weights are introduced for multiview learning to discriminate the contributions of representations from different views. The proposed method outperforms several advanced approaches on semi-supervised classification tasks, demonstrating its feasibility and effectiveness.
关键词:
Multi-view learning, optimization-derived network, representation learning.
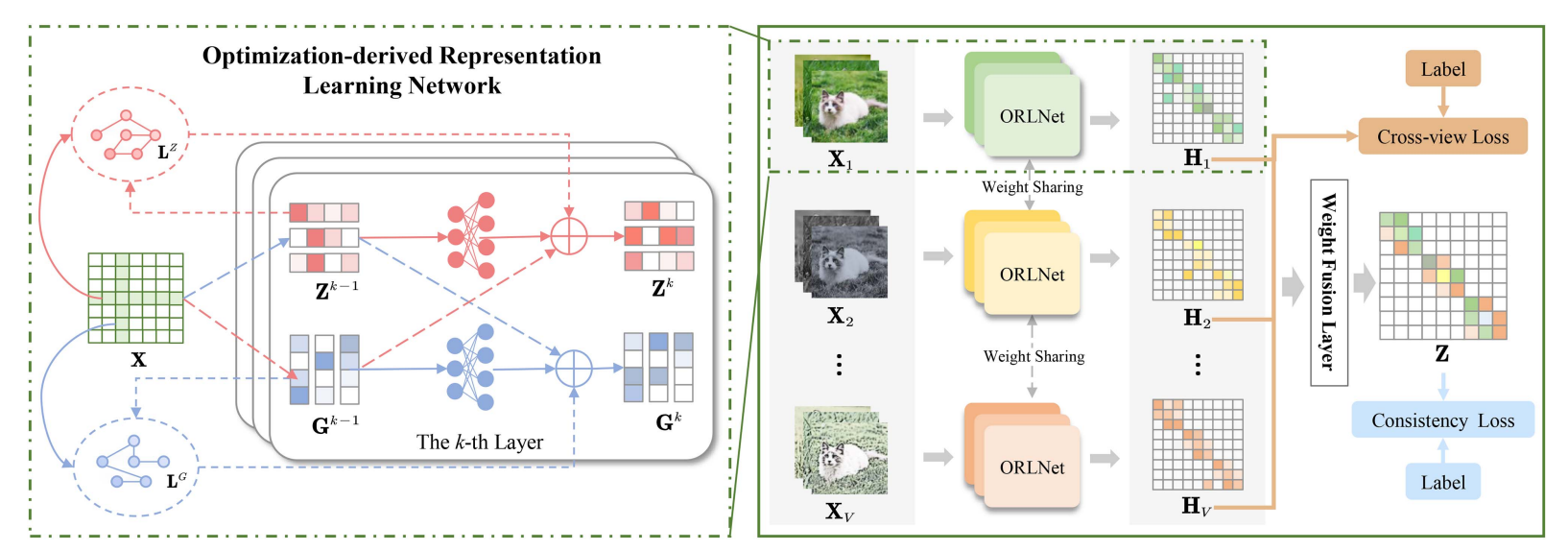