篇名:UMCGL: Universal Multi-view Consensus Graph Learning with Consistency and Diversity
作者:Shide Du , Zhiling Cai , Zhihao Wu , Yueyang Pi, Shiping Wang
年份:2024
DOI:10.1109/TIP.2024.3403055
文章摘要:
Existing multi-view graph learning methods often rely on consistent information for similar nodes within and across views, however they may lack adaptability when facing diversity challenges from noise, varied views, and complex data distributions. These challenges can be mainly categorized into: 1) View-specific diversity within intra-view from noise and incomplete information; 2) Cross-view diversity within inter-view caused by various latent semantics; 3) Cross-group diversity within inter-group due to data distribution differences. To this end, we propose a universal multi-view consensus graph learning framework that considers both original and generative graphs to balance consistency and diversity. Specifically, the proposed framework can be divided into the following four modules: i) Multi-channel graph module to extract principal node information, ensuring view-specific and cross-view consistency while mitigating view-specific and cross-view diversity within original graphs; ii) Generative module to produce cleaner and more realistic graphs, enriching graph structure while maintaining view-specific consistency and suppressing view-specific diversity; iii) Contrastive module to collaborate on generative semantics to facilitate cross-view consistency and reducing cross-view diversity within generative graphs; iv) Consensus graph module to consolidate learning a consensual graph, pursuing cross-group consistency and cross-group diversity. Extensive experimental results on real-world datasets demonstrate its effectiveness and superiority.
关键词:
Multi-view learning, deep learning, graph learning, generative learning, consistency and diversity
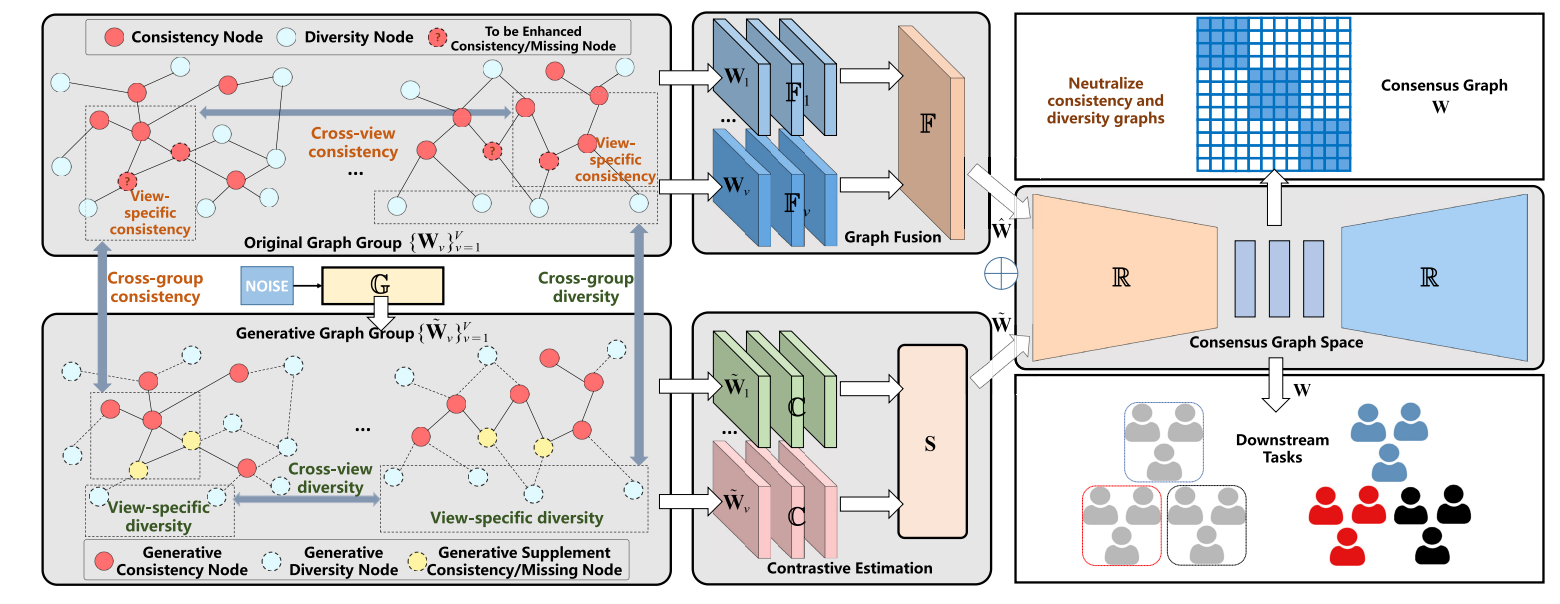