篇名:Unified structure-aware feature learning for Graph Convolutional Network
作者:Sujia Huang, Shunxin Xiao, Yuhong Chen, Jinbin Yang, Zhibin Shi, Yanchao Tan, Shiping Wang
年份:2024
DOI:https://doi.org/10.1016/j.eswa.2024.124397
文章摘要:
Graph Convolutional Network (GCN), as an effective technique for processing graph-structural data, has been widely used in various fields. Label Propagation Algorithm (LPA), also used as a way for message passing on a graph, broadcasts and integrates node labels over the edges. There have been several efforts to explore the joint learning of GCN and LPA for semi-supervised classification tasks. However, they fail to consider the following two points: (1) Capture precise connections and dispel heterophilous relationships between nodes under the mutual supervision of feature and label spaces; (2) Exploit high-order information so that it has varied weight values in affecting distinct nodes. In light of this, we propose a joint framework called structure-aware feature learning for graph convolutional network, which simultaneously takes into account the above two aspects. In specific, the proposed model consists of two modules: feature aggregation and label propagation, aiming to better utilize the information from both label and feature spaces to learn more accurate node representations. As a result, two modules interact with each other to flexibly assign edge weights. Meanwhile, we adopt a structure-aware manner to provide various weights for neighbors of each order of the target node, contributing to optionally capturing high-order information. Experiments indicate that the proposed method outperforms other compared semi-supervised classification models.
关键词:
Graph convolutional network Semi-supervised classification Deep learning Structure learning
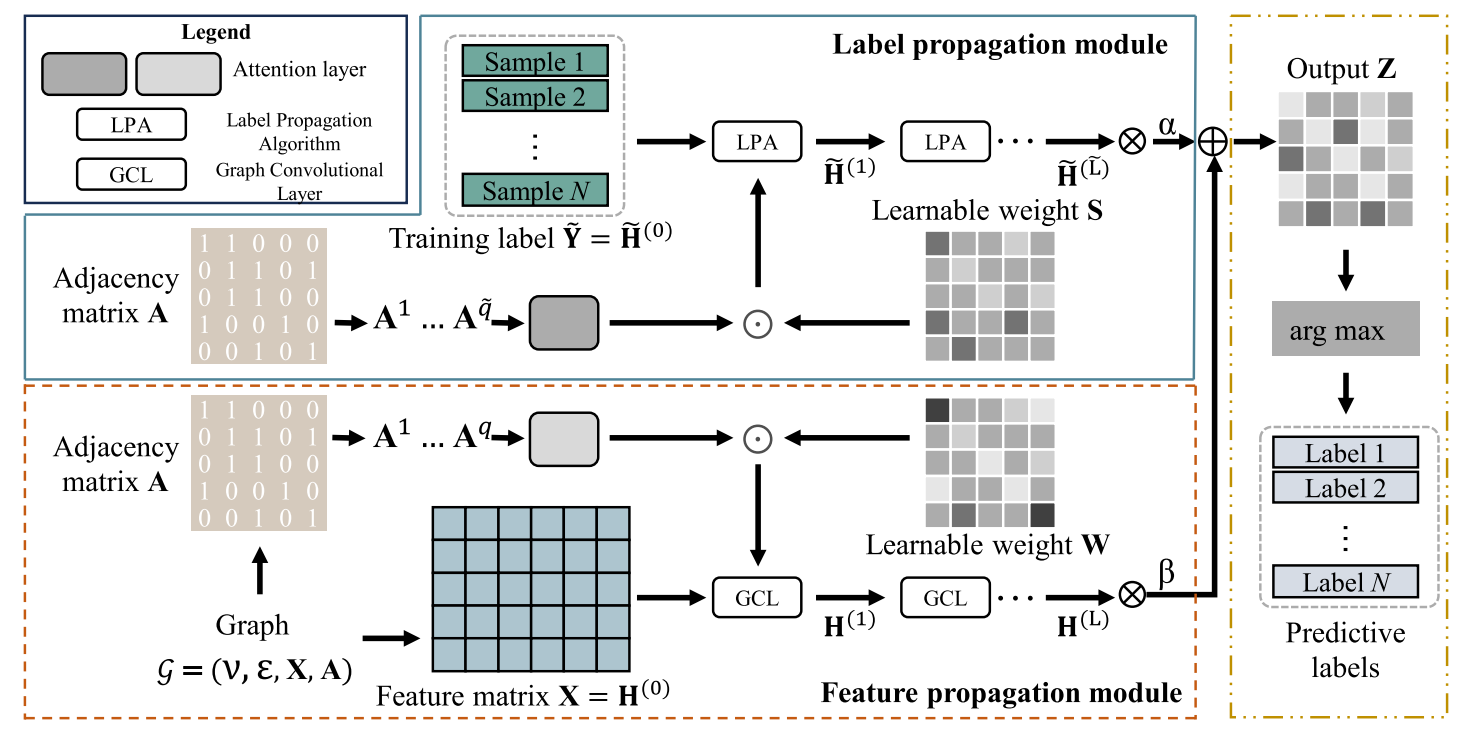