篇名:A Mobile Positioning Method Based on Deep Learning Techniques
作者:Ling Wu, Chi-Hua Chen*(陈志华), Qishan Zhang
来源:Electronics
年份:2019
DOI: 10.3390/electronics8010059
文章摘要:
This study proposes a mobile positioning method that adopts recurrent neural network algorithms to analyze the received signal strength indications from heterogeneous networks (e.g., cellular networks and Wi-Fi networks) for estimating the locations of mobile stations. The recurrent neural networks with multiple consecutive timestamps can be applied to extract the features of time series data for the improvement of location estimation. In practical experimental environments, there are 4525 records, 59 different base stations, and 582 different Wi-Fi access points detected in Fuzhou University in China. The lower location errors can be obtained by the recurrent neural networks with multiple consecutive timestamps (e.g., two timestamps and three timestamps); from the experimental results, it can be observed that the average error of location estimation was 9.19 m by the proposed mobile positioning method with two timestamps.
本研究提出一种移动定位方法,该方法采用递归神经网络算法来分析来自异构网络(例如蜂窝网络和Wi-Fi网络)的接收信号强度指示,以估计移动站的位置。具有多个连续时间戳的递归神经网络可用于提取时间序列数据的特征,以改进位置估计。在实际实验环境中,中国福州大学检测到4525条记录、59个不同的基站和582个不同的Wi-Fi接入点。具有多个连续时间戳(例如,两个时间戳和三个时间戳)的递归神经网络可以获得较低的定位误差;从实验结果可以看出,所提出的具有两个时间戳的移动定位方法的位置估计平均误差为9.19m。
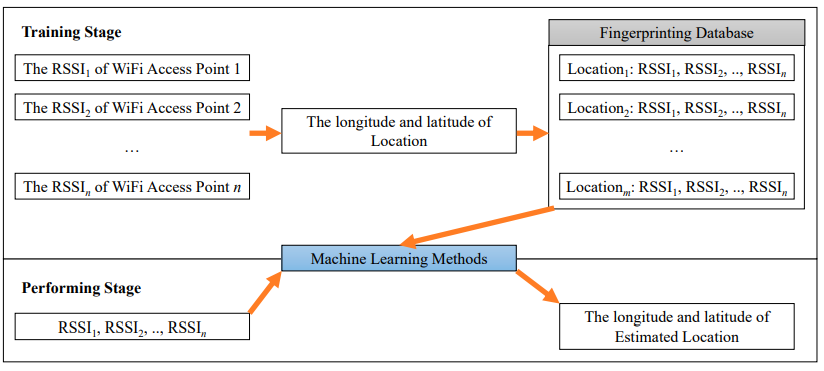
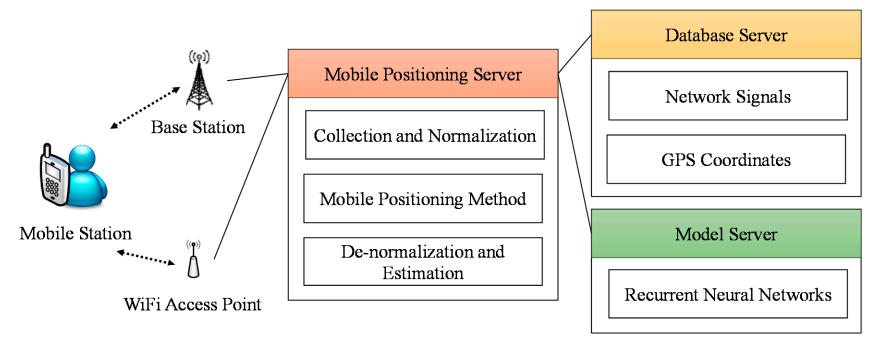