书名:Deep Learning Applications with Practical Measured Results in Electronics Industries
作者:Mong-Fong Horng,Hsu-Yang Kung,Chi-Hua Chen(陈志华),Feng-Jang Hwang
年份:2020
DOI: https://doi.org/10.3390/books978-3-03928-864-9
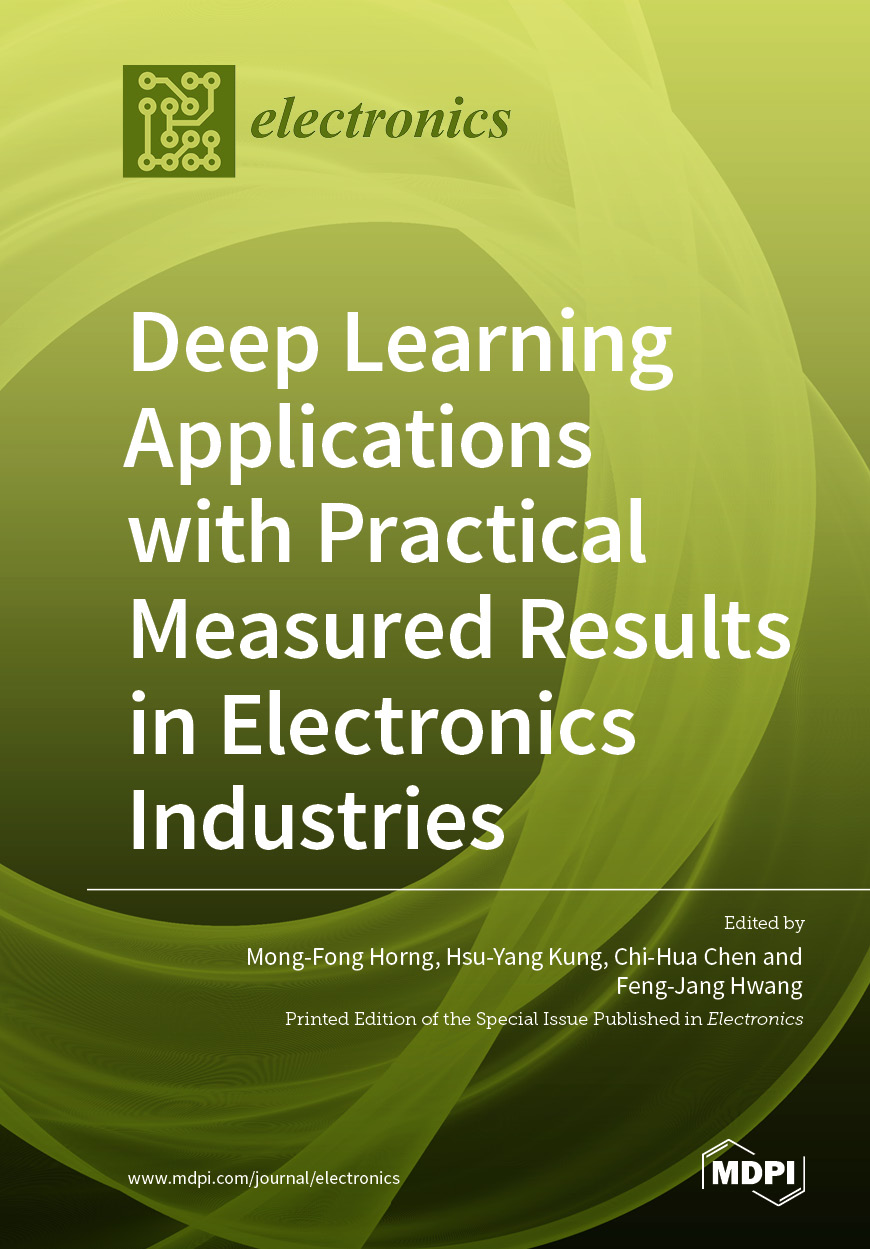
内容简介:Machine learning and deep learning techniques have been the crucial tools when it comes to the feature extracting and event estimating for developing applications in the electronics industries. Some techniques have been implemented in the embedded systems and applied to industry 4.0 applications, industrial electronics applications, consumer electronics applications, and other electronics applications. For instance, supervised learning techniques, including neural networks (NN), convolutional neural networks (CNN), and recurrent neural networks (RNN), can be adopted for prediction applications and classification applications in the electronics industries. Unsupervised learning techniques, including restricted Boltzmann machine (RBM), deep belief networks (DBN), deep Boltzmann machine (DBM), auto-encoders (AE), and denoising auto-encoders (DAE), can be used for denoising and generalization. Furthermore, reinforcement learning techniques, including generative adversarial networks (GANs) and deep Q-networks (DQNs), can be used to obtain generative networks and discriminative networks for contesting and optimizing in a zero-sum game framework. These techniques can provide the precise prediction and classification for electronics applications. Therefore, the aim of this Special Issue is to introduce the readers the state-of-the-art research work on deep learning applications with practical measured results in electronics industries.