篇名:Heterogeneous graph convolutional network for multi-view semi-supervised classification
作者:Shiping Wang, Sujia Huang, Zhihao Wu, Rui Liu, Yong Chen, Dell Zhang
年份:2024
DOI:https://doi.org/10.1016/j.neunet.2024.106438
文章摘要:
This paper proposes a novel approach to semantic representation learning from multi-view datasets, distinct from most existing methodologies which typically handle single-view data individually, maintaining a shared semantic link across the multi-view data via a unified optimization process. Notably, even recent advancements, such as Co-GCN, continue to treat each view as an independent graph, subsequently aggregating the respective GCN representations to form output representations, which ignores the complex semantic interactions among heterogeneous data. To address the issue, we design a unified framework to connect multi-view data with heterogeneous graphs. Specifically, our study envisions multi-view data as a heterogeneous graph composed of shared isomorphic nodes and multi-type edges, wherein the same nodes are shared across different views, but each specific view possesses its own unique edge type. This perspective motivates us to utilize the heterogeneous graph convolutional network (HGCN) to extract semantic representations from multi-view data for semi-supervised classification tasks. To the best of our knowledge, this is an early attempt to transfigure multi-view data into a heterogeneous graph within the realm of multi-view semi-supervised learning. In our approach, the original input of the HGCN is composed of concatenated multi-view matrices, and its convolutional operator (the graph Laplacian matrix) is adaptively learned from multi-type edges in a datadriven fashion. After rigorous experimentation on eight public datasets, our proposed method, hereafter referred to as HGCN-MVSC, demonstrated encouraging superiority over several state-of-the-art competitors for semi-supervised classification tasks.
关键词:
Graph convolutional network Heterogeneous graph Multi-view learning Semi-supervised classification Learnable graph structure
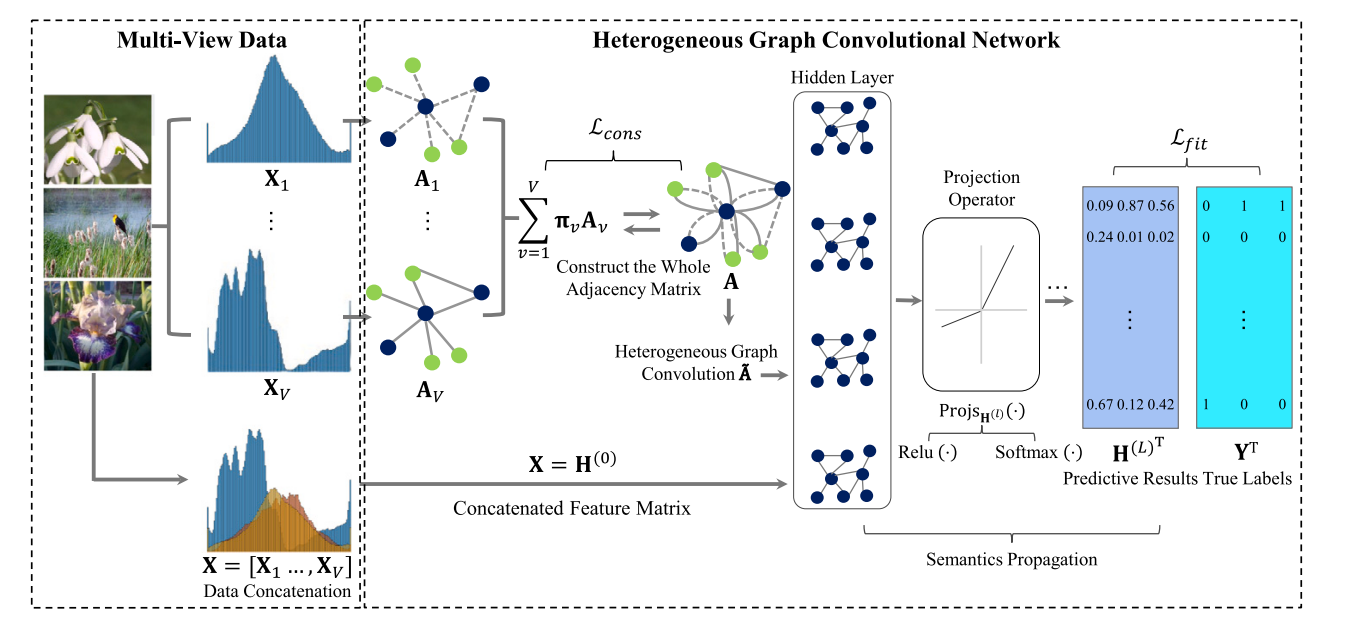