篇名:Tensor-Derived Large-Scale Multi-View Subspace Clustering With Faithful Semantics
作者:Sujia Huang, Shide Du, Lele Fu, Zhihao Wu, Shiping Wang
年份:2024
DOI:10.1109/TSIPN.2024.3414134
文章摘要:
Multi-view subspace clustering is extensively investigated for its ability to extract essential information from multiple data. However, tensor-based methods often encounter several limitations: 1) They suffer from high computational complexity due to the construction of a global affinity matrix; 2) The sophisticated semantic information among samples remains under-explored. To address these issues, we propose a comprehensive framework called tensor-derived large-scale multi-view subspace clustering with faithful semantics, which replaces the original graph with a trustworthy anchor graph. In particular, a graph-optimization-based anchor selection strategy is designed to obtain salient points, and thus the anchor graph is computed to decrease the computational complexity of constructing the representation matrix. Subsequently, a refinement approach is designed to flexibly extract essential semantics between nodes by dividing the graph into significant components and undesired connections. These matrices preserving important information are fused into a tensor that is constrained by a nuclear norm to retain its low-rank property. Meanwhile, the undesired links should be eliminated to avoid confusing the clustering results. Finally, the spectral embedding is employed to directly guide the learning of anchors and graphs. The proposed model achieves a remarkable improvement of 3.3% and 13.1% of ACC on the NoisyMNIST and Prokaryotic datasets while reducing high computational complexity compared to other subspace-based clustering approaches.
关键词:
Multi-view clustering, subspace clustering, lowrank tensor, representation learning, anchor graph.
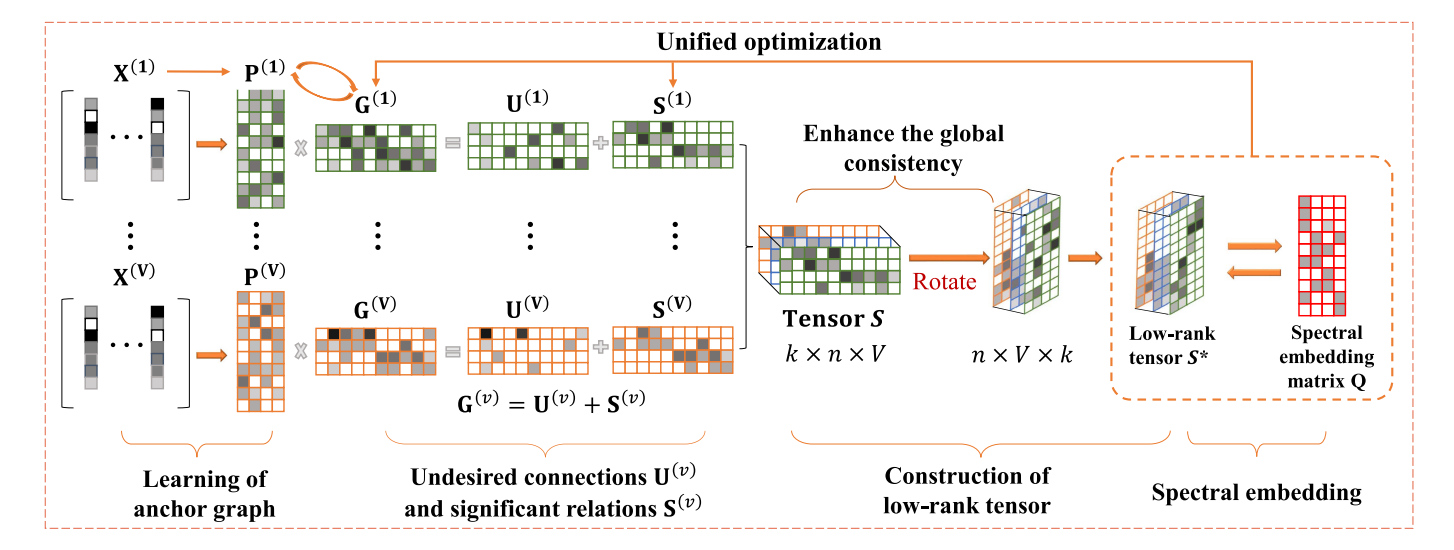