篇名:Adaptive graph active learning with mutual information via policy learning
作者:Yang Huang, Yueyang Pi, Yiqing Shi, Wenzhong Guo, Shiping Wang
年份:2024
DOI:https://doi.org/10.1016/j.eswa.2024.124773
文章摘要:
Graph neural networks entail massive labeled samples for training, and manual labeling generally requires unaffordable costs. Active learning has emerged as a promising approach to selecting a smaller set of informative labeled samples to improve model performance. However, few active learning techniques for graph data account for the cluster structure and redundancy of samples. To address these issues, we propose an approach that employs uncertain information as an observation for a reinforcement learning agent to adaptively learn a node selection policy. We construct states using node information obtained via mutual information, which considers both the graph structure and the node attributes. The proposed method accurately quantifies node information by leveraging the receptive field of the graph convolutional network and capturing the clustering structure of the data, taking into account the low redundancy and diversity of the labeled samples. Experiments conducted on real-world datasets demonstrate the superiority of the proposed approach over several state-of-the-art methods.
关键词:
Active learning Reinforcement learning Deep learning Graph convolutional network Mutual information
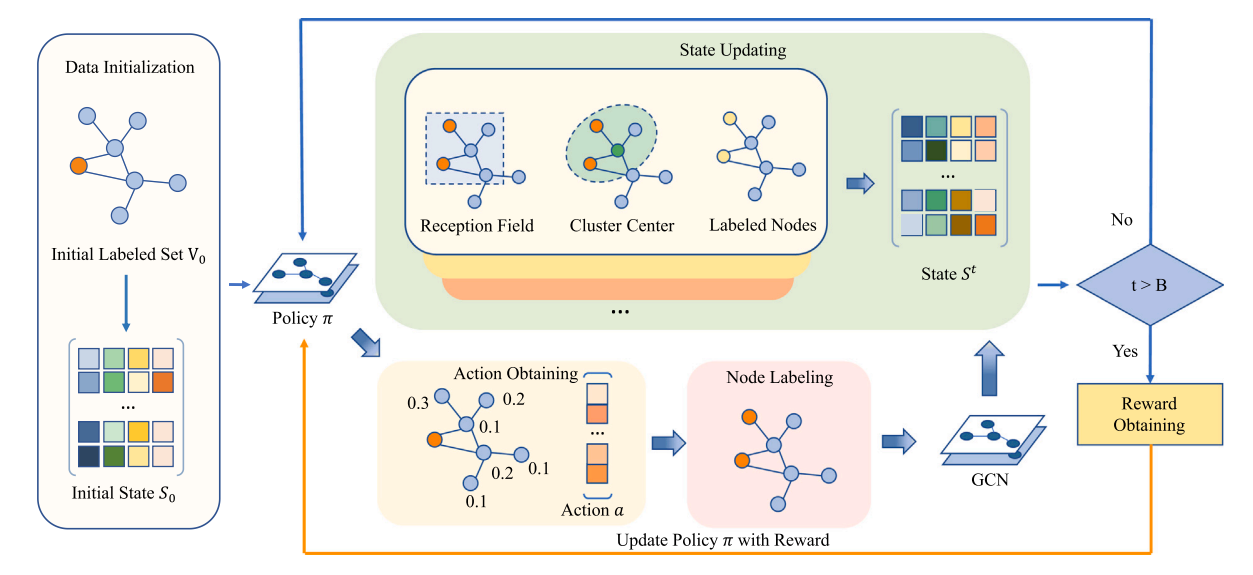