篇名:Inhomogeneous Diffusion-induced Network for Multi-view Semi-supervised Classification
作者:Yueyang Pi, Yilin Wu, Yang Huang, Yongquan Shi, Shiping Wang
年份:2024
DOI:10.1109/TNNLS.2024.3432316
文章摘要:
The challenges posed by heterogeneous data in practical applications have made multiview semi-supervised classification a focus of attention for researchers. While several graph-based approaches have been suggested for this task, they tend to use homogeneous feature propagation, leading to even diffusion of node information to their neighbors. However, this diffusion strategy results in nodes acquiring information of equal proportion from dissimilar samples. In this article, we propose a solution to address these issues by introducing a graph diffusion-induced network for multiview semi-supervised classification. By formulating a discretized partial differential equation on a manifold, we derive a nonlinear and inhomogeneous diffusion equation to govern information propagation on the graph. Then, we investigate the impact of various nonlinear activation functions on random switching edge directions and their suppressive effects on information diffusion between different nodes. In addition, the cross-view consistency under the semi-supervised scenarios is defined and guaranteed for better information fusion. The comprehensive experimental results demonstrate the superiority of the proposed method compared with state-of-the-art approaches. The effectiveness of the proposed approach in handling diverse and heterogeneous data showcases its potential for advancing multiview semi-supervised classification techniques.
关键词:
Graph neural networks, inhomogeneous diffusion, multiview learning, semi-supervised classification.
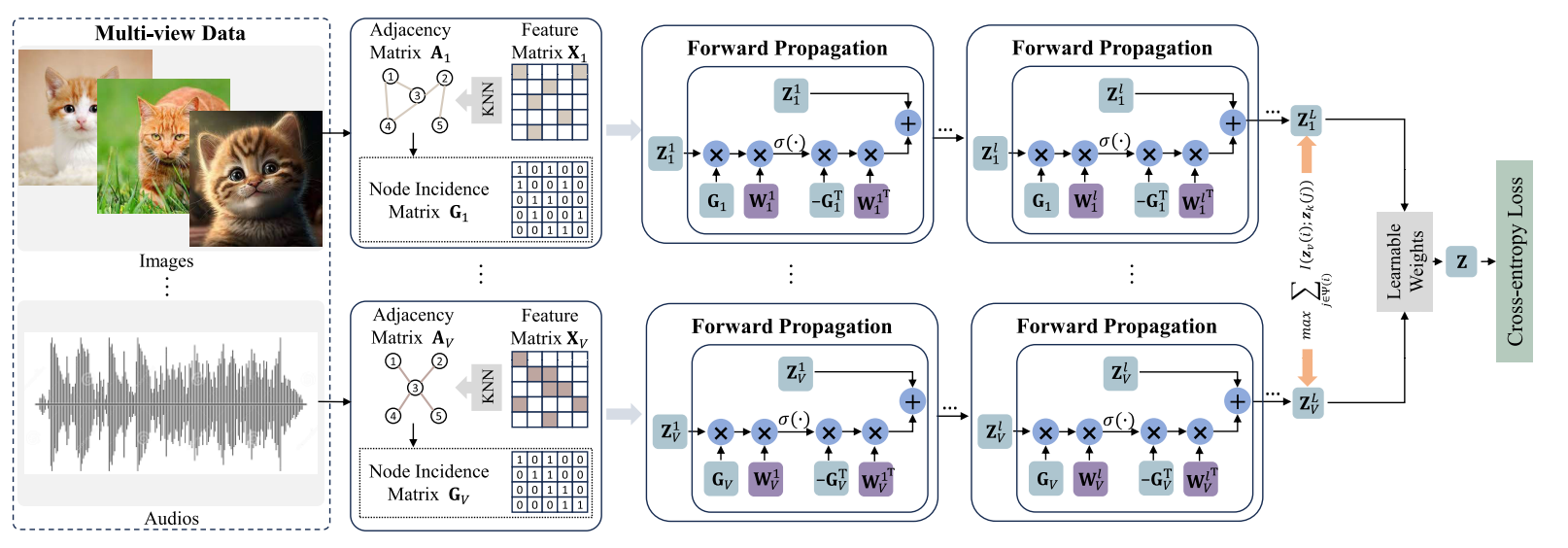