篇名:Water Level Prediction Model Based on GRU and CNN
作者:Mingyang Pan*, Hainan Zhou, Jiayi Cao, Yisai Liu, Jiangling Hao, Shaoxi Li, Chi-Hua Chen(陈志华)
来源:IEEE Access
年份:2020
DOI:10.1109/ACCESS.2020.2973856
文章摘要:
Massive amount of water level data has been collected by using Internet of Things (IoT) techniques in the Yangtze River and other rivers. In this paper, utilizing these data to construct deep neural network models for water level prediction is focused. To achieve higher accuracy, both the factors of time and locations of data collection sensors are considered to perform prediction. And the network structures of gated recurrent unit (GRU) and convolutional neural network (CNN) are combined to build a CNN-GRU model in which the GRU part learns the changing trend of water level, and the CNN part learns the spatial correlation among water level data observed from adjacent water stations. The CNN-GRU model that using data from multiple locations to predict the water level of the middle location has higher accuracy than the model only based on GRU and other state-of-the-art methods including autoregressive integrated moving average model (ARIMA), wavelet-based artificial neural network (WANN) and long-short term memory model (LSTM), because of its ability to decrease the affections of abnormal value and data randomness of a single water station to some extent. The results are verified on an experiment dataset that including 30-year observed data of water level at several collection stations in the Yangtze River. For forecasting the 8-o’clock water levels of future 5 days, accuracy of the CNN-GRU model is better than that of ARIMA, WANN and LSTM models with three evaluation factors including Nash-Sutcliffe efficiency coefficient (NSE), average relative error (MRE) and root mean square error (RMSE).
利用物联网技术在长江和其他河流收集了大量的水位数据。本文主要研究如何利用这些数据建立水位预测的深层神经网络模型。为了获得更高的精度,同时考虑数据采集传感器的时间和位置因素来执行预测。将选通递归单元(GRU)和卷积神经网络(CNN)的网络结构结合起来,建立了一个CNN-GRU模型,其中GRU部分学习水位变化趋势,CNN部分学习相邻水站观测水位数据之间的空间相关性。CNN-GRU模型使用来自多个位置的数据预测中间位置的水位,比仅基于GRU的模型和其他最先进的方法(包括自回归综合移动平均模型(ARIMA)、基于小波的人工神经网络(WANN)和长-短记忆模型(LSTM)具有更高的精度,由于它能够在一定程度上减少单个水站异常值和数据随机性的影响。利用长江多个采集站30年的水位观测数据,对上述结果进行了验证。在预测未来5天8点钟的水位时,CNN-GRU模型的精度优于ARIMA、WANN和LSTM模型,其评估因子包括Nash-Sutcliffe效率系数(NSE)、平均相对误差(MRE)和均方根误差(RMSE)。
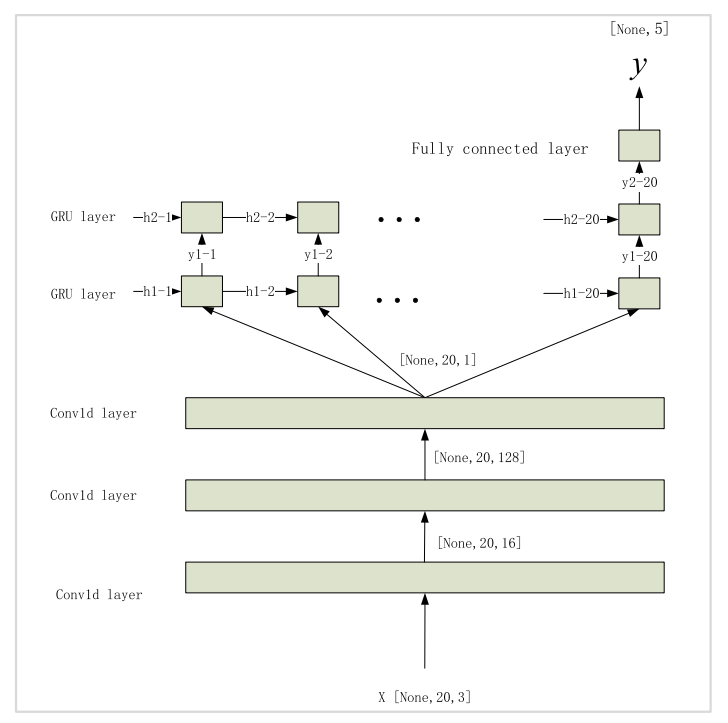