篇名:Differentially Private Knowledge Distillation for Mobile Analytics
作者:Lingjuan Lyu*, Chi-Hua Chen(陈志华)
来源:Proceedings of the 43rd International ACM SIGIR Conference on Research and Development in Information Retrieval (SIGIR 2020), Xi'an, China, July 25th-30th, 2020. (ACM)(CCF Rank A)
年份:2020
DOI: https://doi.org/10.1145/3397271.3401259
文章摘要:
The increasing demand for on-device deep learning necessitates the deployment of deep models on mobile devices. However, directly deploying deep models on mobile devices presents both capacity bottleneck and prohibitive privacy risk. To address these problems, we develop a Differentially Private Knowledge Distillation (DPKD) framework to enable on-device deep learning as well as preserve training data privacy. We modify the conventional Private Aggregation of Teacher Ensembles (PATE) paradigm by compressing the knowledge acquired by the ensemble of teachers into a student model in a differentially private manner. The student model is then trained on both the labeled, public data and the distilled knowledge by adopting a mixed training algorithm. Extensive experiments on popular image datasets, as well as the real implementation on a mobile device show that DPKD can not only benefit from the distilled knowledge but also provide a strong differential privacy guarantee (ε=2$) with only marginal decreases in accuracy.
随着对设备上深度学习需求的不断增长,需要在移动设备上部署深度模型。然而,在移动设备上直接部署deep模型会带来容量瓶颈和禁止性隐私风险。为了解决这些问题,我们开发了一个差异私有知识提取(DPKD)框架,以支持设备上的深度学习并保护培训数据隐私。我们修改了传统的教师集合私有聚合(PATE)范式,将教师集合获得的知识以不同的私有方式压缩到学生模型中。然后,采用混合训练算法,对标记的公共数据和提取的知识对学生模型进行训练。在流行的图像数据集上进行的大量实验以及在移动设备上的实际实现表明,DPKD不仅可以从提取的知识中获益,而且还可以提供强大的差异隐私保证(ε= 2$),且精确度仅略有降低。
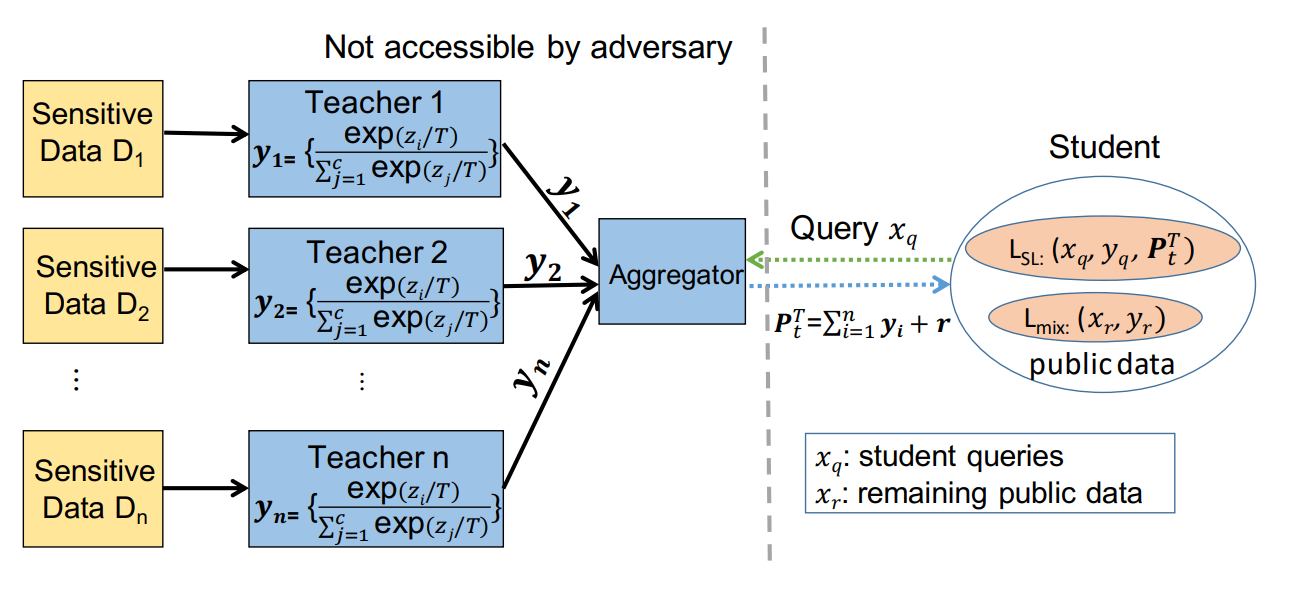