篇名:Matching Sensor Ontologies with Multi-Context Similarity Measure and Parallel Compact Differential Evolution Algorithm
作者:薛醒思(Xingsi Xue),蒋超(Chao Jiang)
来源:IEEE Sensors Journal
年份:2021
DOI: 10.1109/JSEN.2021.3115471
文章摘要:
Sensor ontology is able to resolve the sensor data heterogeneity issue among the Cybertwin-driven 6G based Internet of Everything (IoE) systems. However, due to human subjectivity, the sensor ontologies also suffer from the heterogeneity problem. To address this problem, it is necessary to execute the sensor Ontology Matching (OM) process, i.e., finding the identical entity pairs between two ontologies. To this end, we first propose a Multi-Context based ESM (MC-ESM) to measure the similarity of two entities by taking into consideration their semantic contexts. After that, a parallel compact Differential Evolution with Adaptive Step Length (pcDE-ASL) is proposed to find all entity mappings, which uses the probability presentation on the population to save the memory consumption, and the parallel processing mechanism and ASL to help the algorithm efficiently converge on the global optima. The experimental results show that pcDE-ASL is both effective and efficient.
为了解决传感器本体异质问题,本文提出了一种基于多上下文的相似度技术,通过考虑两个实体的语义上下文来度量它们的相似性。在此基础上,提出了一种自适应步长的并行紧致差分进化算法(pcDE-ASL)来寻找所有实体映射,该算法利用种群的概率表示来节省内存消耗,并利用并行处理机制和适应性步长来帮助算法高效地收敛于全局最优解。实验结果表明,pcDE-ASL是一种有效且高效的方法。
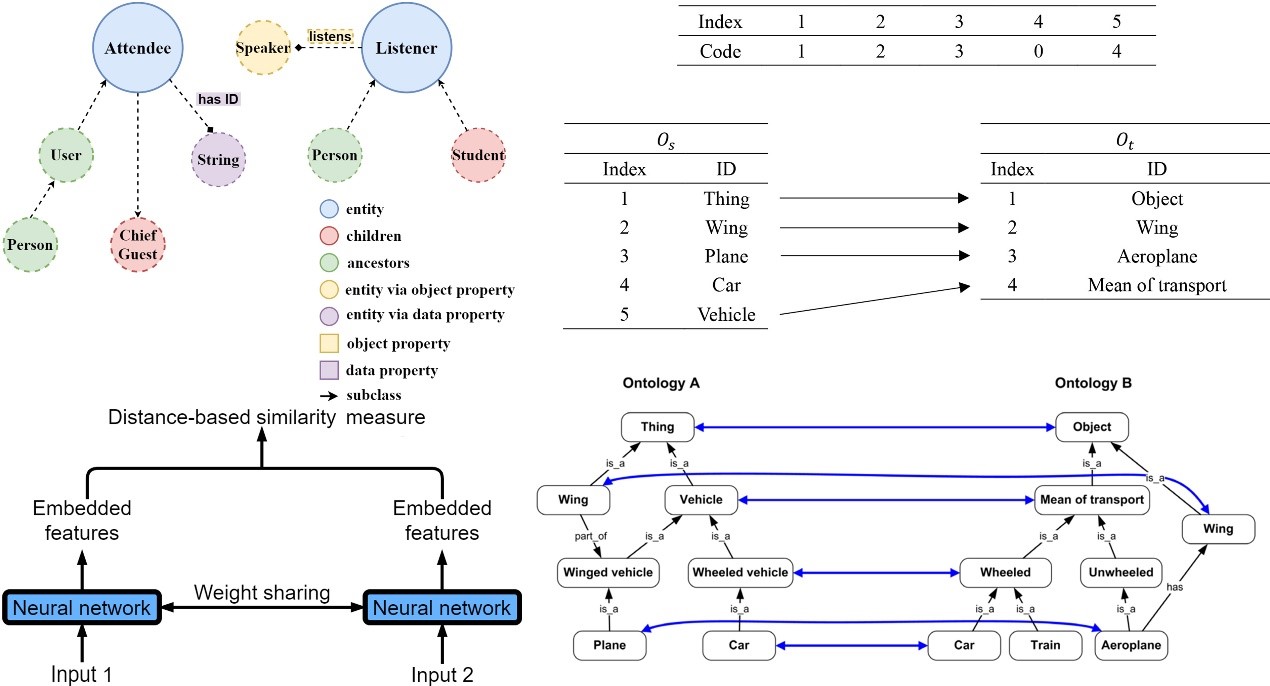